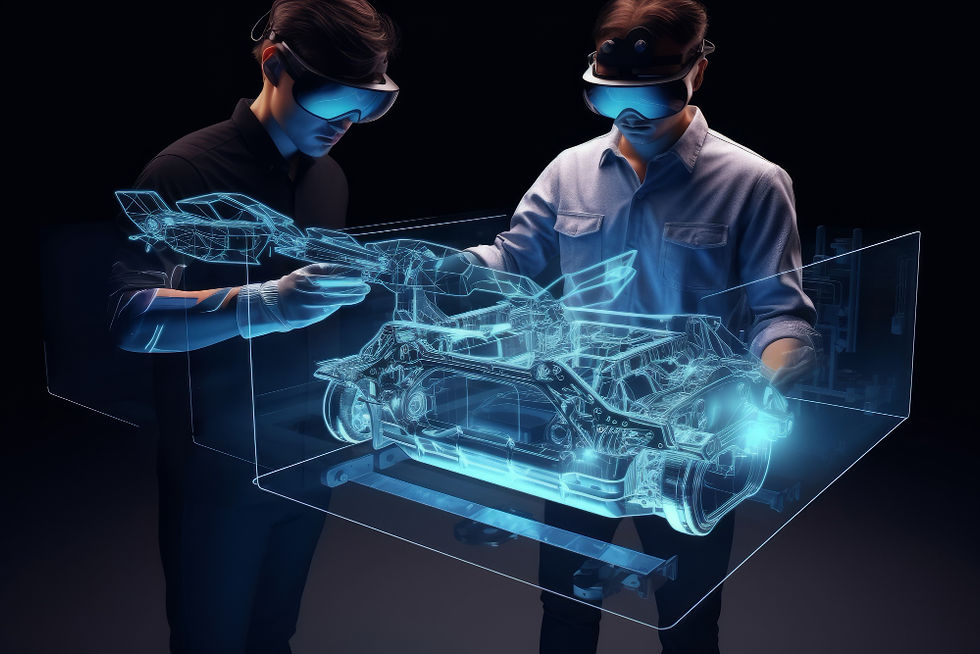
Autonomous testing leverages automation and AI to conduct testing processes independently, relying heavily on high-quality data and marketing technology to produce accurate and efficient results. Data quality plays a crucial role in ensuring the reliability and effectiveness of autonomous testing.
Understanding Data Quality in Autonomous Testing
In the context of autonomous testing, data quality refers to the accuracy, completeness, consistency, and timeliness of the data used for training and testing AI models. High data quality is essential for reliable and effective testing processes, as it directly impacts the outcomes and performance of AI models.
Significance of Data Quality in Autonomous Testing
Poor data quality can lead to biased outcomes and inaccurate conclusions in autonomous testing, affecting the performance and reliability of AI models. For example, biased data can result in skewed predictions and flawed testing results, compromising the overall effectiveness of the testing process.
Strategies for Data Management in Autonomous Testing
Data Collection and Cleaning
Collecting accurate and representative data is crucial for training AI models effectively. Cleaning and organizing data help maintain high data quality, ensuring that the AI models perform optimally during testing.
Monitoring and Maintenance
Ongoing monitoring is essential to uphold high data quality standards over time. Regular maintenance of data sets enhances the effectiveness of autonomous testing by ensuring that the data remains relevant and reliable for testing purposes.
Addressing Common Challenges
Challenges such as inconsistent data, bias, inadequate test data coverage, and data privacy can be mitigated through strategies like data preprocessing, bias detection algorithms, comprehensive test data generation, and robust data privacy protocols. These measures help reduce biases and ensure comprehensive test coverage in autonomous testing.
Human Element in Data Management
Human testers play a critical role in identifying and managing risks associated with data quality in autonomous testing. Human oversight is essential for validating AI-generated results, ensuring accuracy, and maintaining the integrity of the testing process.
Conclusion
“Data quality is paramount in autonomous testing, influencing the reliability and effectiveness of AI models. Organizations should prioritize data quality and implement robust data management strategies to achieve successful autonomous testing outcomes. By emphasizing data quality and employing effective data management practices, organizations can enhance the performance and accuracy of autonomous testing processes, as highlighted in leading b2b tech publications.”
コメント